An in-depth look at the multi-faceted skillset required to excel in the dynamic field of data science.
Data science has captivated interest with its magical promise of extracting valuable insights from data.
However, behind the scenes, data science is an intricate field requiring broad, multidisciplinary expertise.
The Allure of Data Science
What makes data science so appealing?
- Derive actionable intelligence – Discover hidden patterns to predict outcomes accurately.
- Automate manual tasks – Systems leveraging AI can self-learn and improve over time.
- Get ahead of the competition – Data-driven decisions give a strategic advantage.
However, misconceptions abound about its ease of access.
Evaluating the Data Science Skill Stack
Mastering data science requires rigorous effort – understanding models is just the foundation.
As a data science practitioner on a data science agency team, my journey highlights key competencies:
Technical Capabilities
- Mathematics and statistics – To comprehend complex algorithms.
- Coding skills – To operationalize theoretical concepts into working models with Python, SQL, etc.
- Data engineering – To gather, clean, transform, and pipeline data at scale.
- Machine learning – To build, optimize, and productionize predictive models.
Business Focus
- Intuitive communication – To contextualize and convey technical insights simply.
- Domain experience – To anchor data initiatives into real-world sectors and use cases.
- Strategy alignment – To tie goals back to tangible business outcomes.
Demystifying the “Black Box” of Data Science
Many fixate on the tip of the iceberg – visualizing future trends with AI seems almost magical!
Inside the Black Box
But the bulk hidden underneath drives this wizardry:
- Data ingestion – API integrations, web scraping.
- Data preprocessing – Cleaning, transforming, feature engineering.
- Model optimization – Statistical analysis, machine learning fine-tuning.
- Deployment and monitoring – Containerization, CI/CD pipelines.
This entire machine powers the glamorous insights data science generates. Mastering the mechanics within the black box is far from trivial.
The Road Ahead: Perpetual Learning
Data science intersects multiple domains – technology, analytics, business strategy. What’s cutting edge today becomes obsolete tomorrow.
Expanding Horizons
Additional facets continue shaping modern data science:
- Ethics – Identifying and minimizing bias in data.
- Transparency – Balancing model interpretability vs performance.
- Privacy – Adhering to evolving regulations around data.
- Sustainability – Optimizing energy efficiency in computation.
Lifelong active learning is imperative to stay relevant as data science continues its exponential growth trajectory.
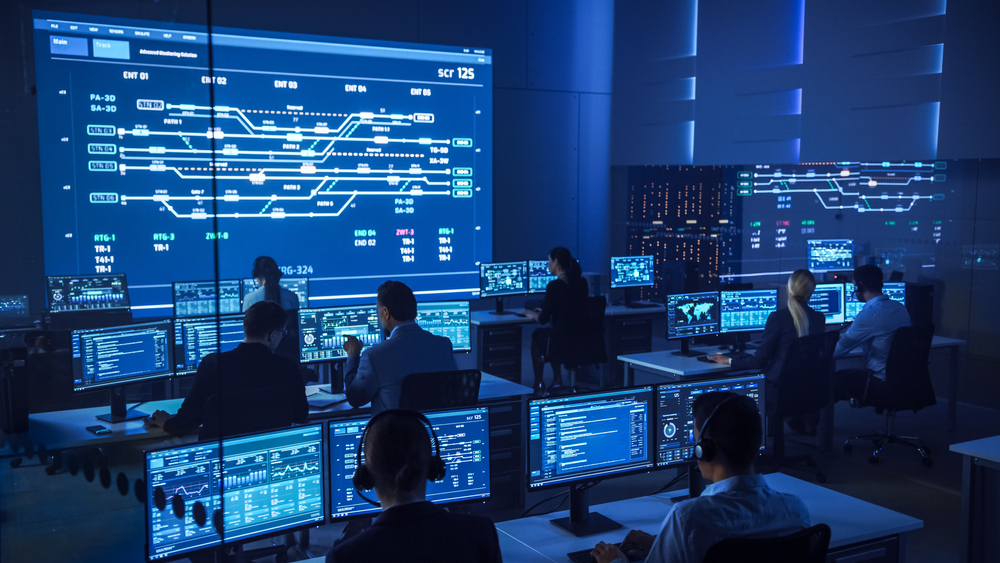
Conclusion: The Magic Has Its Price
Behind data science’s dazzling facade lies tremendous complexity powering its innovation. Mastery demands intellectual rigor across diverse areas – technology, communication, business – along with perpetual learning.
However, magic always comes at a price! For those curious enough to peek behind the curtain and work hard at honing these multidimensional skills, data science promises rich rewards in terms of knowledge, growth, and impact. So while data science continues to intrigue us with its wizardry, the tremendous effort required to sustain its spell is reserved only for those enterprising enough to pay its price!